The 11th IAPR International Conference on Biometrics (ICB2018) was held on Feb. 20-23, 2018 in Gold Coast, Australia. ICB is sponsored by IAPR (Technical Committee on Biometrics – TC4, International Association for Pattern Recognition), and is the premier forum for the presentation of new advances and research results in the fields of biometrics. ICB 2018 attracts more than 120 scholars and professional persons all around the world, including the world leaders in biometric field such as Prof. Anil K. Jain and Prof. Arun Ross from Michigan State University in US, and Prof. Josef Kittler from University of Surrey in UK. This conference is hosted by Prof. Brian Lovell from University of Queensland and Prof. Jun Zhou from Griffith University.
Prof. Shiguang Shan gave a tutorial report at this conference. The title of the tutorial report is "Face Recognition in Deep Learning: Recent Progress and Some Trends". In this speech, Prof. Shan introduced the impact of depth learning on the field of face recognition, the application of face recognition in China, and the technological innovations behind it. This speech attracted the largest number of participants in all tutorial reports. The discussions were very active.
In addition, Prof. Shan also presented VIPL’s latest research paper in improving RGB face recognition with the use of RGBD face data. Its title is "improving 2D face recognition via discriminative face estimation" with Jiyun Cui, Hao Zhang, Hu Han, Shiguang Shan and Xilin Chen as co-authors. With the popularity of depth cameras, there are more and more RGBD (3D) databases, but many practical face recognition applications can only use RGB (2D) cameras for face recognition. This paper proposes a discriminative face depth estimation approach to improve 2D face recognition accuracies under unconstrained scenarios. The proposed discriminative depth estimation method uses a cascaded FCN and CNN architecture, in which FCN aims at recovering the depth from an RGB image, and CNN retains the separability of individual subjects. The estimated depth information is then used as a complementary modality to RGB for face recognition tasks. Experiments on two public datasets and a dataset we collect show that the proposed face recognition method using RGB and estimated depth information can achieve better accuracy than using RGB modality alone.
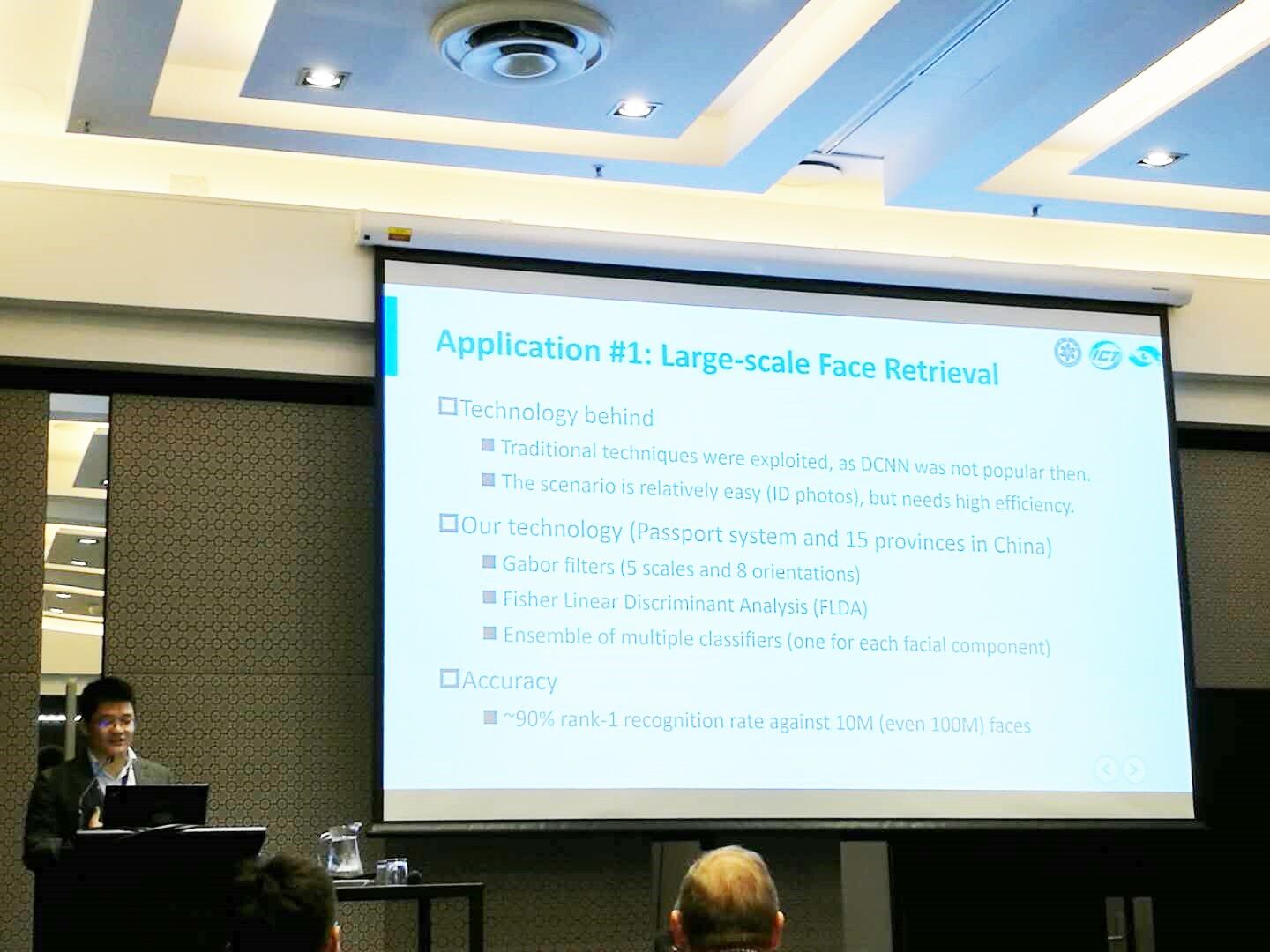