Congratulations! ECCV2020 shows that there are VIPL's 7 papers are accepted! ECCV is the top European conference in the image analysis area. ECCV2020 will be held in Glasgow, United Kingdom, in August 2020.
1. Sketching Image Gist: Human-Mimetic Hierarchical Scene Graph Generation (Wenbin Wang, Ruiping Wang, Shiguang Shan, Xilin Chen)
Scene graph aims to faithfully reveal humans’ perception of image content. It is not generated just for being admired, but for supporting downstream tasks, such as image captioning, where a description is supposed to depict the major event in the image, or the namely image gist. When humans analyze a scene, they usually prefer to describe image gist first. This humans’ inherent perceptive habit implies that there exists a hierarchical structure about humans’ preference during the scene parsing procedure. Therefore, we argue that a desirable scene graph should be also hierarchically constructed, and introduce a new scheme for modeling scene graph.
Concretely, a scene is represented by a human-mimetic Hierarchical Entity Tree (HET) consisting of a series of image regions. To generate a scene graph based on HET, we parse HET with a Hybrid Long Short-Term Memory (Hybrid-LSTM) which specifically encodes hierarchy and siblings context to capture the structured information embedded in HET. To further prioritize key relations in the scene graph, we devise a Relation Ranking Module (RRM) to dynamically adjust their rankings by learning to capture humans’ subjective perceptive habits from objective entity saliency and size. Experiments indicate that our method not only achieves state-of-the-art performances for scene graph generation, but also is expert in mining image-specific relations which play a great role in serving downstream tasks.
2. Temporal Complementary Learning for Video Person Re-Identification (Ruibing Hou, Hong Chang, Bingpeng Ma, Shiguang Shan, Xilin Chen)
This paper presents a Temporal Complementary Learning Network that extracts complementary features for consecutive video frames. Firstly, we propose a Temporal Saliency Erasing (TSE) module including a saliency erasing operation and a series of ordered learners. Specifically, for a specific frame of a video, the saliency erasing operation drives the specific learner to mine new and complementary parts by erasing the parts activated by previous frames. Such that the diverse visual features can be discovered for consecutive frames and finally form an integral characteristic of the target identity. Furthermore, a Temporal Saliency Boosting (TSB) module is designed to propagate the salient information among video frames to enhance the salient feature. It is complementary to TSE by effectively alleviating the information loss caused by the erasing operation of TSE. Extensive experiments show our method is a simple and effective framework and performs favorably against state-of-the-arts.
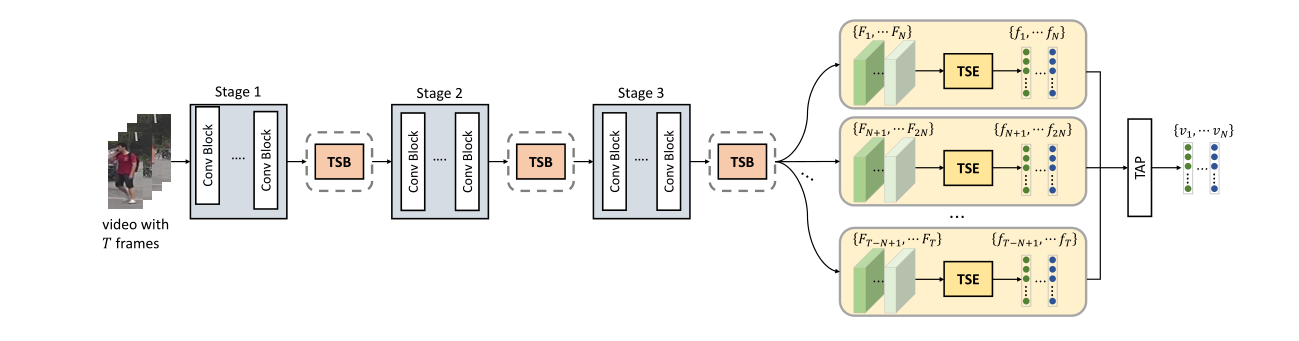
3. Appearance-Preserving 3D Convolution for Video-based Person Re-identification (Xinqian Gu, Hong Chang, Bingpeng Ma, Hongkai Zhang, Xilin Chen)
Due to the imperfect person detection results and posture changes, temporal appearance misalignment is unavoidable in video based person re-identification (ReID). In this case, 3D convolution may destroy the appearance representation of person video clips, thus it is harmful to ReID. To address this problem, we propose Appearance Preserving 3D Convolution (AP3D), which is composed of two components: an Appearance-Preserving Module (APM) and a 3D convolution kernel. With APM aligning the adjacent feature maps in pixel level, the following 3D convolution can model temporal information on the premise of maintaining the appearance representation quality. It is easy to combine AP3D with existing 3D ConvNets by simply replacing the original 3D convolution kernels with AP3Ds. Extensive experiments demonstrate the effectiveness of AP3D for video-based ReID and the results on three widely used datasets surpass the state-of-the-arts.
Fig. 1: Temporal appearance misalignment caused by (a) smaller bounding boxes, (b) bigger bounding boxes and (c) posture changes. (d) AP3D firstly uses APM to reconstruct the adjacent feature maps to guarantee the appearance alignment with respect to the central feature map and then performs 3D convolution.
4. Dynamic R-CNN: Towards High Quality Object Detection via Dynamic Training (Hongkai Zhang, Hong Chang, Bingpeng Ma, Naiyan Wang, Xilin Chen)
Although two-stage object detectors have continuously advanced the state-of-the-art performance in recent years, the training process itself is far from crystal. In this work, we first point out the inconsistency problem between the fixed network settings and the dynamic training procedure, which greatly affects the performance. Then, we propose Dynamic R-CNN to adjust the label assignment criteria (IoU threshold) and the shape of regression loss function (parameters of SmoothL1 Loss) automatically based on the statistics of proposals during training. This dynamic design makes better use of the training samples and pushes the detector to _t more high quality samples. Specifically, our method improves upon ResNet-101-FPN base-line with 2.0% AP and 6.1% AP90 on the MS COCO dataset with no extra overhead.
Fig 2. Dynamic R-CNN Pipeline
5. Video-based Remote Physiological Measurement via Cross-verified Feature Disentangling. (Xuesong Niu, Zitong Yu, Hu Han, Xiaobai Li, Shiguang Shan, Guoying Zhao)
Remote physiological measurements, e.g., remote photoplethysmography (rPPG) based heart rate (HR), heart rate variability (HRV) and respiration frequency (RF) measuring, are playing more and more important roles under the application scenarios where contact measurement is inconvenient or impossible. Since the amplitude of the physiological signals is very small, they can be easily affected by head movements, lighting conditions, and sensor diversities. To address these challenges, we propose a cross-verified feature disentangling strategy to disentangle the physiological features with non-physiological representations, and then use the distilled physiological features for robust multi-task physiological measurements. We first transform the input face videos into a multi-scale spatial-temporal map (MSTmap), which can suppress the irrelevant background and noise features while retaining most of the temporal characteristics of the periodic physiological signals. Then we take pairwise MSTmaps as inputs to an autoencoder architecture with two encoders (one for physiological signals and the other for non-physiological information) and use a cross-verified scheme to obtain physiological features disentangled with the non-physiological features. The disentangled features are finally used for the joint prediction of multiple physiological signals like average HR values and rPPG signals. Comprehensive experiments on different large-scale public datasets of multiple physiological measurement tasks as well as the cross-database testing demonstrate the robustness of our approach. This work received consistent positive recommendation from all the reviewers, and has been selected as oral presentation for ECCV2020 (top 2%, 104 orals /5025 submissions).
6. Object-Contextual Representations for Semantic Segmentation. (Yuhui Yuan, Xilin Chen, Jingdong Wang)
In this paper, we study the context aggregation problem in semantic segmentation. Motivated by that the label of a pixel is the category of the object that the pixel belongs to, we present a simple yet effective approach, object-contextual representations, characterizing a pixel by exploiting the representation of the corresponding object class. First, we learn object regions under the supervision of the ground-truth segmentation. Second, we compute the object region representation by aggregating the representations of the pixels lying in the object region. Last, we compute the relation between each pixel and each object region, and augment the representation of each pixel with the object-contextual representation which is a weighted aggregation of all the object region representations. We empirically demonstrate our method achieves competitive performance on various benchmarks: Cityscapes, ADE20K, LIP, PASCAL-Context and COCO-Stuff. Our submission “HRNet + OCR + SegFix” achieves the 1st place on the Cityscapes leaderboard by the ECCV 2020 submission deadline.
Fig 3. Pipeline of Object Contextual Representation
7. SegFix: Model-Agnostic Boundary Refinement for Segmentation. (Yuhui Yuan, Jingyi Xie, Xilin Chen, Jingdong Wang)
We present a model-agnostic post-processing scheme to improve the boundary quality for the segmentation result that is generated by any existing segmentation model. Motivated by the empirical observation that the label predictions of interior pixels are more reliable, we propose to replace the originally unreliable predictions of boundary pixels by the predictions of interior pixels. Our approach processes only the input image through two steps: (i) localize the boundary pixels and (ii) identify the corresponding interior pixel for each boundary pixel. We build the correspondence by learning a direction away from the boundary pixel to an interior pixel. Our method requires no prior information of the segmentation models and achieves nearly real-time speed. We empirically verify that our SegFix consistently reduces the boundary errors for segmentation results generated from various state-of-the-art models on Cityscapes, ADE20K and GTA5.
Fig 4. Pipeline of SegFix