Congratulations! ICCV2019 shows that there are VIPL's 5 papers are accepted! ICCV is a top international conference on computer vision, pattern recognition and artificial intelligence hosted by IEEE. ICCV2019 will be held in Seoul, Korea, in October 2019.
1. Adaptive Reconstruction Network for Weakly Supervised Referring Expression Grounding (Xuejing Liu, Liang Li, Shuhui Wang, Zheng-Jun Zha, Dechao Meng, Qingming Huang)
Weakly supervised referring expression grounding aims at localizing the referential object in an image according to the linguistic query, where the mapping between the referential object and query is unknown in the training stage. To address this problem, we propose a novel end-to-end adaptive reconstruction network (ARN). It builds the correspondence between image region proposal and query in an adaptive manner: adaptive grounding and collaborative reconstruction. Specifically, we first extract the subject, location and context features to represent the proposals and the query respectively. Then, we design the adaptive grounding module to compute the matching score between each proposal and query by a hierarchical attention model. Finally, based on attention score and proposal features, we reconstruct the input query with a collaborative loss of language reconstruction loss, adaptive reconstruction loss, and attribute classification loss. This adaptive mechanism helps our model to alleviate the variance of different referring expressions. Experiments on four large-scale datasets show ARN outperforms existing state-of-the-art methods by a large margin. Qualitative results demonstrate that the proposed ARN can better handle the situation where multiple objects of a particular category situated together.
2. Transferable Contrastive Network for Generalized Zero-Shot Learning (Huajie Jiang, Ruiping Wang, Shiguang Shan, Xilin Chen)
Zero-Shot learning (ZSL) studies the problem of recognizing new categories which have no labeled images for training, where the key is utilizing seen-class images to learn the knowledge and transferring the knowledge to novel classes. Most existing approaches learn the knowledge by space transformation among seen classes and they are probable to encounter the domain shift problem so they perform badly in generalized zero-shot learning (GZSL). To tackle such problem, we propose the transferable contrastive network (TCN), which learns the contrastive mechanism between images and class semantics for image recognition. It explicitly transfers the knowledge from seen-class images to the novel classes by class similarities in the training process. Therefore, TCN could effectively alleviate the domain shift problem and it is more suitable to novel categories. Extensive experiments on four widely-used ZSL datasets (AWA, APY, CUB, SUN) show the effectiveness of the proposed approach for both ZSL and GZSL.
3. Weakly Supervised Object Detection with Segmentation Collaboration (Xiaoyan Li, Meina Kan, Shiguang Shan, Xilin Chen)
Weakly supervised object detection aims at learning precise object detectors, given image category labels. In recent prevailing works, this problem is generally formulated as a multiple instance learning module guided by an image classification loss. The object bounding box is assumed to be the one contributing most to the classification among all proposals. However, the region contributing most is also likely to be a crucial part or the supporting context of an object. To obtain a more accurate detector, in this work we propose a novel end-to-end weakly supervised detection approach, where a newly introduced generative adversarial segmentation module interacts with the conventional detection module in a collaborative loop. The collaboration mechanism takes full advantages of the complementary interpretations of the weakly supervised localization task, namely detection and segmentation tasks, forming a more comprehensive solution. Consequently, our method obtains more precise object bounding boxes, rather than parts or irrelevant surroundings. Expectedly, the proposed method achieves an accuracy of 50.2% with a single-stage model on the PASCAL VOC 2007 dataset, outperforming the state-of-the-arts and demonstrating its superiority for weakly supervised object detection.
4. S2GAN: Share Aging Factors across Ages and Share Aging Trends among Individuals (Zhenliang He, Meina Kan, Shiguang Shan, Xilin Chen)
Generally, we human follow the roughly common aging trends, e.g., the wrinkles only tend to be more, longer or deeper. However, the aging process of each individual is more dominated by his/her personalized factors, including the invariant factors such as identity and mole, as well as the personalized aging patterns, e.g., one may age by graying hair while another may age by receding hairline. Following this biological principle, in this work, we propose an effective and efficient method to simulate natural aging. Specifically, a “personalized aging basis” is established for each individual to depict his/her own aging factors. Then different ages share this basis, being derived through “age-specific transforms”. The age-specific transforms represent the aging trends which are shared among all individuals. The proposed method can achieve continuous face aging with favorable aging accuracy, identity preservation, and fidelity. Furthermore, befitted from the effective design, a unique model is capable of all ages and the prediction time is significantly saved.
5. Temporal Knowledge Propagation for Image-to-Video Person Re-identification (Xinqian Gu, Bingpeng Ma, Hong Chang, Shiguang Shan, Xilin Chen)
In many scenarios of Person Re-identification, the gallery set consists of lots of surveillance videos and the query is just an image, thus Re-ID has to be conducted between image and videos. Compared with videos, still person images lack temporal information. Besides, the information asymmetry between image and video features increases the difficulty in matching images and videos. To solve this problem, we propose a novel Temporal Knowledge Propagation method which propagates the temporal knowledge learned by the video representation network to the image representation network. Specifically, given the input videos, we enforce the image representation network to fit the outputs of video representation network in a shared feature space. With back propagation, temporal knowledge can be transferred to enhance the image features and the information asymmetry problem can be alleviated. With additional classification and integrated triplet losses, our model can learn expressive and discriminative image and video features for image-to-video re-identification. Extensive experiments demonstrate the effectiveness of our method and the overall results on two widely used datasets surpass the state-of-the-art methods by a large margin.
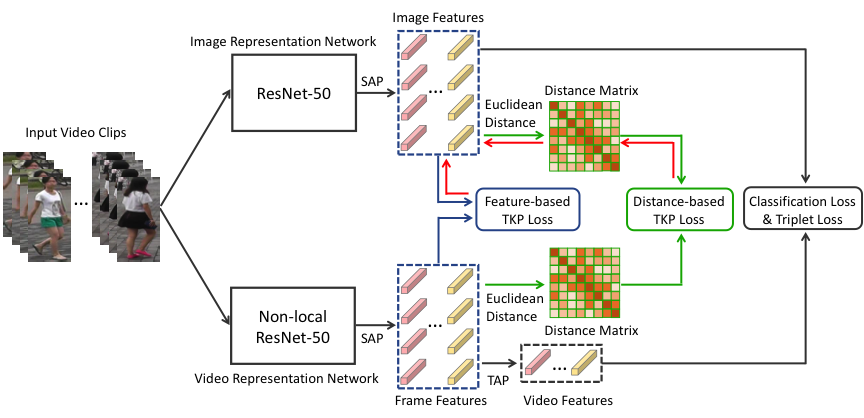
Download: