For person re-identification, the feature generated for a pedestrian sequence is often corrupted by the mis-detected frames. In addition, different identities may have similar local parts which are difficult to distinguish the two persons. In this work, we propose to leverage the spatial-temporal context information to clarify the local distractions to enhance the target feature representation. Specifically, we present a novel block, Interaction-Aggregation-Update (IAU) block. As shown in the figure below, we firstly use a part division unit to extract the part features for each frame. Then we perform interaction and aggregation to the part features. We consider two types of relations: spatial relations and temporal relations. Here the spatial relation learns to compute the contextual dependencies between different body parts in a single frame. While the temporal relation is used to capture the contextual dependencies between same body parts across all frames. With help of the spatial and temporal contexts, the features of the corrupted parts can be adaptively changed to describe the target person. The proposed IAU block is lightweight, end-to-end trainable and can be easily plugged into existing CNNs to form the IAUnet. We conduct extensive experiments on both image and video based reID benchmarks. Experimental results show that our IAUnet performs favorably against state-of-the-arts on five reID datasets.
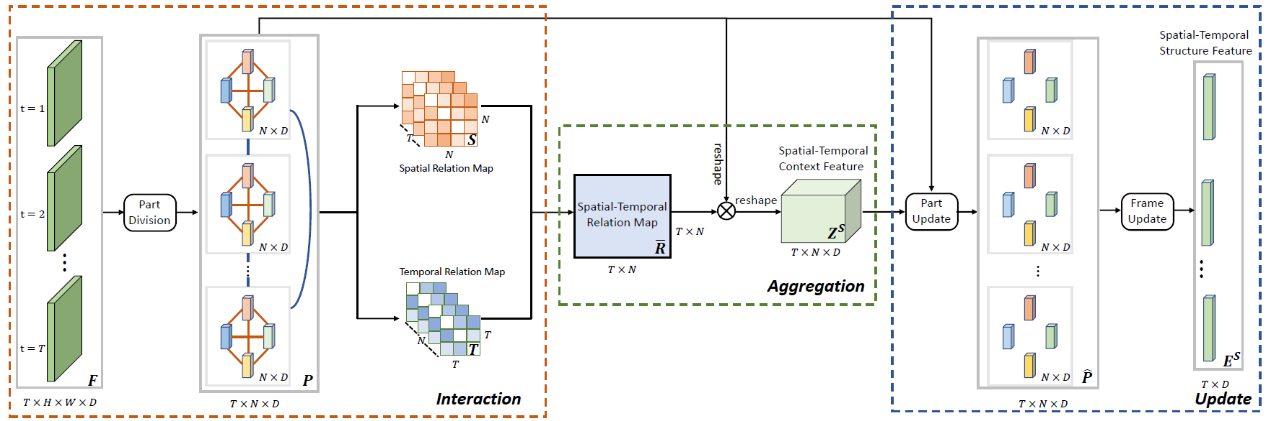
Download: